[ad_1]
Disclaimer: This is a machine generated PDF of selected content from our products. This functionality is provided solely for your convenience and is in no way intended to replace original scanned PDF. Neither Cengage Learning nor its licensors make any representations or warranties with respect to the machine generated PDF. The PDF is automatically generated “AS IS” and “AS AVAILABLE” and are not retained in our systems. CENGAGE LEARNING AND ITS LICENSORS SPECIFICALLY DISCLAIM ANY AND ALL EXPRESS OR IMPLIED WARRANTIES, INCLUDING WITHOUT LIMITATION, ANY WARRANTIES FOR AVAILABILITY, ACCURACY, TIMELINESS, COMPLETENESS, NON-INFRINGEMENT, MERCHANTABILITY OR FITNESS FOR A PARTICULAR PURPOSE. Your use of the machine generated PDF is subject to all use restrictions contained in The Cengage Learning Subscription and License Agreement and/or the Gale College Collection Terms and Conditions and by using the machine generated PDF functionality you agree to forgo any and all claims against Cengage Learning or its licensors for your use of the machine generated PDF functionality and any output derived therefrom.
What are you talking about? Meaning and machine intelligence Date: Jan. 7, 2017 From: The Economist(Vol. 422, Issue 9022) Publisher: Economist Intelligence Unit N.A. Incorporated Document Type: Article Length: 1,658 words
Full Text: Machines still cannot conduct proper conversations with humans because they do not understand the world
IN “BLACK MIRROR”, a British science-fiction satire series set in a dystopian near future, a young woman loses her boyfriend in a car accident. A friend offers to help her deal with her grief. The dead man was a keen social-media user, and his archived accounts can be used to recreate his personality. Before long she is messaging with a facsimile, then speaking to one. As the system learns to mimic him ever better, he becomes increasingly real.
This is not quite as bizarre as it sounds. Computers today can already produce an eerie echo of human language if fed with the appropriate material. What they cannot yet do is have true conversations. Truly robust interaction between man and machine would require a broad understanding of the world. In the absence of that, computers are not able to talk about a wide range of topics, follow long conversations or handle surprises.
Machines trained to do a narrow range of tasks, though, can perform surprisingly well. The most obvious examples are the digital assistants created by the technology giants. Users can ask them questions in a variety of natural ways: “What’s the temperature in London?” “How’s the weather outside?” “Is it going to be cold today?” The assistants know a few things about users, such as where they live and who their family are, so they can be personal, too: “How’s my commute looking?” “Text my wife I’ll be home in 15 minutes.”
And they get better with time. Apple’s Siri receives 2bn requests per week, which (after being anonymised) are used for further teaching. For example, Apple says Siri knows every possible way that users ask about a sports score. She also has a delightful answer for children who ask about Father Christmas. Microsoft learned from some of its previous natural-language platforms that about 10% of human interactions were “chitchat”, from “tell me a joke” to “who’s your daddy?”, and used such chat to teach its digital assistant, Cortana.
The writing team for Cortana includes two playwrights, a poet, a screenwriter and a novelist. Google hired writers from Pixar, an animated-film studio, and The Onion, a satirical newspaper, to make its new Google Assistant funnier. No wonder people often thank their digital helpers for a job well done. The assistants’ replies range from “My pleasure, as always” to “You don’t need to thank me.”
Good at grammar
How do natural-language platforms know what people want? They not only recognise the words a person uses, but break down speech for both grammar and meaning. Grammar parsing is relatively advanced; it is the domain of the well-established field of “natural-language processing”. But meaning comes under the heading of “natural-language understanding”, which is far harder.
First, parsing. Most people are not very good at analysing the syntax of sentences, but computers have become quite adept at it, even though most sentences are ambiguous in ways humans are rarely aware of. Take a sign on a public fountain that says, “This is not drinking water.” Humans understand it to mean that the water (“this”) is not a certain kind of water (“drinking water”). But a computer might just as easily parse it to say that “this” (the fountain) is not at present doing something (“drinking water”).
As sentences get longer, the number of grammatically possible but nonsensical options multiplies exponentially. How can a machine parser know which is the right one? It helps for it to know that some combinations of words are more common than others: the phrase “drinking water” is widely used, so parsers trained on large volumes of English will rate those two words as likely to be joined in a noun phrase. And some structures are more common than others: “noun verb noun noun” may be much more common than “noun noun verb noun”. A machine parser can compute the overall probability of all combinations and pick the likeliest.
A “lexicalised” parser might do even better. Take the Groucho Marx joke, “One morning I shot an elephant in my pyjamas. How he got in my pyjamas, I’ll never know.” The first sentence is ambiguous (which makes the joke)–grammatically both “I” and “an elephant” can attach to the prepositional phrase “in my pyjamas”. But a lexicalised parser would recognise that “I [verb phrase] in my pyjamas” is far more common than “elephant in my pyjamas”, and so assign that parse a higher probability.
But meaning is harder to pin down than syntax. “The boy kicked the ball” and “The ball was kicked by the boy” have the same meaning but a different structure. “Time flies like an arrow” can mean either that time flies in the way that an arrow flies, or that insects called “time flies” are fond of an arrow.
“Who plays Thor in ‘Thor’?” Your correspondent could not remember the beefy Australian who played the eponymous Norse god in the Marvel superhero film. But when he asked his iPhone, Siri came up with an unexpected reply: “I don’t see any movies matching ‘Thor’ playing in Thor, IA, US, today.” Thor, Iowa, with a population of 184, was thousands of miles away, and “Thor”, the film, has been out of cinemas for years. Siri parsed the question perfectly properly, but the reply was absurd, violating the rules of what linguists call pragmatics: the shared knowledge and understanding that people use to make sense of the often messy human language they hear. “Can you reach the salt?” is not a request for information but for salt. Natural-language systems have to be manually programmed to handle such requests as humans expect them, and not literally.
Multiple choice
Shared information is also built up over the course of a conversation, which is why digital assistants can struggle with twists and turns in conversations. Tell an assistant, “I’d like to go to an Italian restaurant with my wife,” and it might suggest a restaurant. But then ask, “is it close to her office?”, and the assistant must grasp the meanings of “it” (the restaurant) and “her” (the wife), which it will find surprisingly tricky. Nuance, the language-technology firm, which provides natural-language platforms to many other companies, is working on a “concierge” that can handle this type of challenge, but it is still a prototype.
Such a concierge must also offer only restaurants that are open. Linking requests to common sense (knowing that no one wants to be sent to a closed restaurant), as well as a knowledge of the real world (knowing which restaurants are closed), is one of the most difficult challenges for language technologies.
Common sense, an old observation goes, is uncommon enough in humans. Programming it into computers is harder still. Fernando Pereira of Google points out why. Automated speech recognition and machine translation have something in common: there are huge stores of data (recordings and transcripts for speech recognition, parallel corpora for translation) that can be used to train machines. But there are no training data for common sense.
Knowledge of the real world is another matter. AI has helped data-rich companies such as America’s West-Coast tech giants organise much of the world’s information into interactive databases such as Google’s Knowledge Graph. Some of the content of that appears in a box to the right of a Google page of search results for a famous figure or thing. It knows that Jacob Bernoulli studied at the University of Basel (as did other people, linked to Bernoulli through this node in the Graph) and wrote “On the Law of Large Numbers” (which it knows is a book).
Organising information this way is not difficult for a company with lots of data and good AI capabilities, but linking information to language is hard. Google touts its assistant’s ability to answer questions like “Who was president when the Rangers won the World Series?” But Mr Pereira concedes that this was the result of explicit training. Another such complex query–“What was the population of London when Samuel Johnson wrote his dictionary?”–would flummox the assistant, even though the Graph knows about things like the historical population of London and the date of Johnson’s dictionary. IBM’s Watson system, which in 2011 beat two human champions at the quiz show “Jeopardy!”, succeeded mainly by calculating huge numbers of potential answers based on key words by probability, not by a human-like understanding of the question.
Making real-world information computable is challenging, but it has inspired some creative approaches. Cortical.io, a Vienna-based startup, took hundreds of Wikipedia articles, cut them into thousands of small snippets of information and ran an “unsupervised” machine-learning algorithm over it that required the computer not to look for anything in particular but to find patterns. These patterns were then represented as a visual “semantic fingerprint” on a grid of 128×128 pixels. Clumps of pixels in similar places represented semantic similarity. This method can be used to disambiguate words with multiple meanings: the fingerprint of “organ” shares features with both “liver” and “piano” (because the word occurs with both in different parts of the training data). This might allow a natural-language system to distinguish between pianos and church organs on one hand, and livers and other internal organs on the other.
Proper conversation between humans and machines can be seen as a series of linked challenges: speech recognition, speech synthesis, syntactic analysis, semantic analysis, pragmatic understanding, dialogue, common sense and real-world knowledge. Because all the technologies have to work together, the chain as a whole is only as strong as its weakest link, and the first few of these are far better developed than the last few.
The hardest part is linking them together. Scientists do not know how the human brain draws on so many different kinds of knowledge at the same time. Programming a machine to replicate that feat is very much a work in progress.
What machines cannot yet do is have true conversations
Copyright: COPYRIGHT 2017 Economist Intelligence Unit N.A. Incorporated http://store.eiu.com/
Source Citation (MLA 8th Edition) “What are you talking about? Meaning and machine intelligence.” The Economist, vol. 422, no. 9022, 7 Jan. 2017, p. 9(US). Gale
College Collection, link.gale.com/apps/doc/A476622738/GCCO?u=mia56118&sid=lms-GCCO&xid=214bf16b. Accessed 23 June 2021.
Gale Document Number: GALE|A476622738
[Button id=”1″]
"96% of our customers have reported a 90% and above score. You might want to place an order with us."
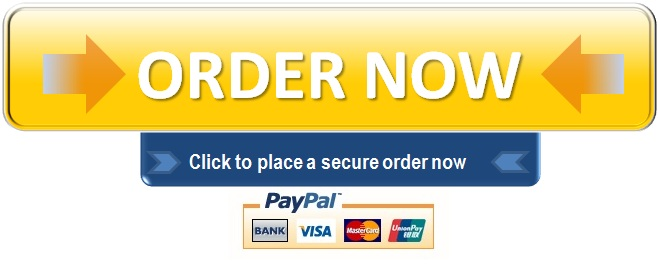